To combat gender bias in AI, organizations should implement bias detection algorithms, utilize balanced training data, foster diverse AI teams, ensure transparency, conduct regular audits, follow ethical guidelines, offer sensitivity training, aim for continuous improvement, make gender equality a design goal, and engage with varied stakeholders. Continuous revisiting and stakeholder feedback are essential for equitable AI systems.
Leading Contributors for This Article
Implement Bias Detection and Correction Algorithms
Organizations can ensure their AI systems are free of gender bias by developing and integrating algorithms specifically designed to detect and correct bias. These algorithms can scrutinize data for patterns of gender bias and adjust the AI's learning process to minimize it, ensuring fairer outcomes.
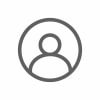
Utilize Diverse Training Data Sets
One key step is to use diverse and balanced training data sets that accurately represent all genders. An inclusive data set helps prevent the AI system from developing skewed perspectives that favor one gender over others, promoting equality in automated decisions.
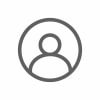
Promote Gender Diversity Among AI Teams
Organizations should strive for gender diversity among the teams that design, develop, and deploy AI systems. Diverse teams are more likely to recognize potential biases and take steps to mitigate them, leading to more equitable AI solutions.
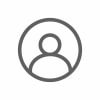
Adopt Transparency in AI Operations
Increasing transparency in how AI systems operate and make decisions can help identify and eliminate gender biases. Letting stakeholders understand the decision-making process enables them to pinpoint bias and advocate for necessary changes.
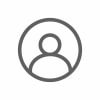
Regular Bias Audits
Conducting regular audits of AI systems to check for gender bias is crucial. Independent audits can uncover unintentional biases that have crept into the system, allowing organizations to take corrective action promptly.
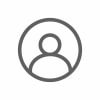
Incorporate Ethical AI Guidelines
Adopting ethical AI guidelines that specifically address gender bias can guide the development and deployment of AI systems. These guidelines can serve as a benchmark for ensuring gender equity is a central consideration in all AI initiatives.
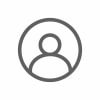
Training on Gender Sensitivity for AI Practitioners
Offering gender sensitivity training for developers and AI practitioners can raise awareness about the potential for bias in AI systems. Educated teams are better equipped to recognize and combat gender biases in their work.
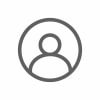
Continuous Improvement Process
Organizations should view the elimination of gender bias in AI as a continuous improvement process rather than a one-time fix. By constantly revising and updating AI systems, they can respond to new insights about gender bias and refine their approaches over time.
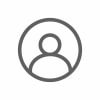
Explicitly Model Gender Equality as a Goal
Incorporating gender equality as an explicit goal in AI system design can ensure that the final product reflects these values. Designing algorithms with gender equity as a core objective helps prioritize the elimination of biases from the outset.
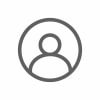
Engage with Stakeholders
Engaging with a broad range of stakeholders, including those from underrepresented genders, can provide valuable insights into how AI systems might perpetuate gender biases. Stakeholder feedback can inform more nuanced and comprehensive approaches to creating equitable AI.
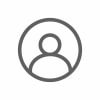
What else to take into account
This section is for sharing any additional examples, stories, or insights that do not fit into previous sections. Is there anything else you'd like to add?
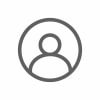
Contribute to three or more articles across any domain to qualify for the Contributor badge.